Hazardous Asteroid Classification with Machine Learning using Physical and Orbital Asteroid Properties
DOI:
https://doi.org/10.58445/rars.49Keywords:
Potentially Hazardous Asteroids, Machine Learning, Random Forest ClassificationAbstract
Asteroids, rocky objects orbiting the sun, have been a key focus of scientific study as they can provide insights into planet formation. With a seemingly infinite number of asteroids in space, the possibility of one colliding with our planet and leading to devastating effects constantly looms large. Asteroids that could come in close proximity or collide with earth are classified as potentially hazardous asteroids, PHA (NASA, n.d.). However, it becomes cumbersome for humans to manually analyze large datasets for identifying all the possibly dangerous asteroids. Thus, machine learning techniques are ideal to study trends and make predictions. Machine learning is a method of data analysis based on computer algorithms that model relationships and improve our ability to analyze asteroid threats. It has been applied to automate the asteroid classification process in the past, for instance by Anish Si in 2018 at the Vellore Institute of Technology in India, where his 15-tree Random Forest model performed the best (Si, 2018). The goal of this study was to train multiple machine learning models on physical and orbital asteroid features and identify the model that most accurately classified the asteroids as hazardous or non-hazardous. The key enhancements were that a different subset of features and significantly different list of models were used for classification. The results showed that a 50-tree Random Forest classification model had a 98.45% accuracy on the test set validating that the Random Forest is the most optimal model for asteroid classification.
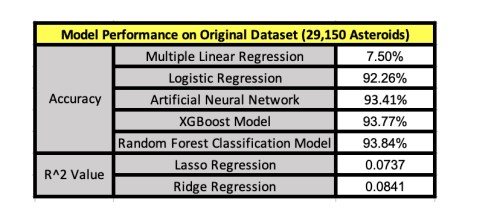
Downloads
Posted
Versions
- 2022-12-22 (3)
- 2022-12-22 (2)
- 2022-11-01 (1)
Categories
License
Copyright (c) 2022 Arjun Ramakrishnan

This work is licensed under a Creative Commons Attribution-NonCommercial-NoDerivatives 4.0 International License.